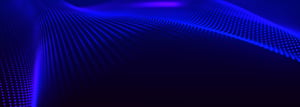
Understand how our AI engine works under the hood
Click on the images below to explore how we harness data and transform it into powerful investment decisions:
Understanding the clients' investiment needs
At Axyon AI we focus on developing the
best deep learning solutions to boost
clients' investment
strategies.
Hence, our very first
step is to understand clients' needs in
regards to the investment objective like
the investment universe, the investment
horizon, the benchmark and the risk
management constraints and anything else
that needs to be considered.
Data research
Our database is continuously updated with new high-quality predictive and alternative sources of data.
Why is it
important?
This step is
important to ensure a structured data
research process as new, and original
data sources can improve alpha
generation thanks to our AI models*.
*AI modelling is the creation of a logical decision-making system based on a certain set of available data.
Contact usDataset preparation
Data is at the centre of every AI engine. And data preparation is one of the most critical phases.
It starts with collecting data such as stock prices, fundamental data, indexes futures, currencies, interest rates, macroeconomic indicators, analysts' estimates, and technical indicators from our data providers.
During the data preparation step, our Quants and Data Engineers validate, identify and rectify inconsistencies, and deal with outliers, anomalies, structural changes and any missing data. After the data preparation, the data is clean and ready to be transformed and engineered to create features.
Why is it
important?
Data
preparation helps us to get the most out
of the data. In this step, the quality
and the quantity of data influence
directly how good our predictive model
will be. Without this phase, we can end
up spending a lot of time looking at bad
AI results. The better the data, the
more outstanding the results.
What is the
output?
Output: clean
datasets, ready to be used to train the
AI models.
In our case, for each
investment product (Equities, Futures,
Commodities), there is a different
dataset containing features.
Ai model development
INTRO: This step may be considered the heart of the Axyon IRIS technology stack.
đź’ˇIf our products were cars, this is the step where we produce the engines. Here, our Machine Learning Team will use our proprietary AutoML engine as a factory to train and optimize forecasting models, based on multiple state-of-the-art AI technologies.
More specifically, the clean dataset generated in the previous step will be used to train supervised machine learning models with the goal to forecast the ranking of target financial assets in terms of expected return.
đź’ˇThis is analogous to how you would slowly learn a new language by attempting to translate short random sentences and receiving feedback from a teacher: you would slowly learn what works and what doesn't, and gradually improve at this task.
During the training, each ML model learns how to rank financial assets based on the expected relative performance over a given time horizon after processing millions of historical data points. Besides optimizing individual models, our Platform is able to automatically tune hyperparameters** and perform feature selection, thanks to advanced Evolutionary Computation*** techniques.
**Hyperparameter tuning means adjusting the parameters that the model cannot learn and need to be provided before training.
***Evolutionary Computation (EC) is the technique used in complex optimization on problems that have too many variables for traditional algorithms to consider.
Models are then evaluated in terms of proprietary metrics assessing both their accuracy and, crucially, robustness to several scenarios that may occur. Finally, an ensemble of the best models is automatically created and deployed to our model registry for live usage. We also generate out-of-sample historical predictions for unbiased model evaluation and analysis in this step.
Processing large amounts of data is very computationally demanding. Therefore, our Platform relies on a flexible and scalable workload management system which automatically summons accelerated computation nodes on the cloud (supporting multiple providers) or on connected HPC (high-performance computer) resources.
Why is it
important?
This is
important for two reasons:
(i) a big part of Axyon's R&D effort is dedicated to the improvement of financial AI modelling, hence this step is where our technology really shines;
(ii) the resulting AI models and their forecasting ability will be one of the key ingredients in the construction of our AI-powered investment strategies in later phases.
Once trained, our AI models will be ready
to answer the key
question:
"How will asset
XYZ perform over the next month
compared to other
assets?"
How long does this phase
take?
In general,
training ML models can take from seconds
on a laptop to months on a large-scale
GPU cluster. It depends on the
complexity of the model and dataset.
In our case, it may take from a few days up to about a week, depending on the size of the target investment universe.
Our Machine Learning Team is actively working to improve the ML model training process, with the goal of training more accurate models, faster.
What is the
output?
This step
produces optimised AI-based forecasting
models, which will be deployed as a key
component of the end product.
Ai-signal analysis
After the model deployment, our proprietary AutoML engine produces AI-predictive signals that allow investors to access AI algorithms insights.
The signals are transformed into rankings based on the assets' predictive performances given a time horizon. Those AI signals are then monitored and analysed to determine whether or not they have helped address the client's business needs.
Why is it
important?
This is
important to evaluate the overall
quality and stability of the signals and
to understand the relationship between
known investing factors and ML signals.
What is the
output?
Output: reports
that contain detailed information about
the historical signals
Ai rankings & ai-powered strategies
The final outputs of the model discovery process are relative performance rankings that can be used to build different investment strategies (according to the client's requirements).
These rankings identify predicted outperformers and underperformers within a given investment universe and time horizon.
The first way to integrate AI into an existing process is directly trading a fully-fledged AI-based strategy.
To build such a strategy, an investor could transform the daily ranking into target portfolio weights that guide the portfolio allocation. As an example, a portfolio manager could choose to create a long-only strategy that every week puts equal weight on each of the top 30 assets of the AI-generated ranking.
A different alternative to integrating AI into an existing process is treating AI signals and rankings as an alternative alpha-generating data source. This approach can be illustrated using predictive rankings for overweight or underweight assets already traded by discretionary asset managers.
Contact us
AI solutions for enhanced investment management
Axyon AI is, first and foremost, a technology company. Our team of engineers, scientists and quantitative researchers is on a mission to build a cutting-edge technology stack for AI-based time series modelling.
OUR PARTNERS
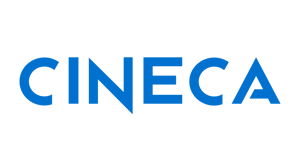
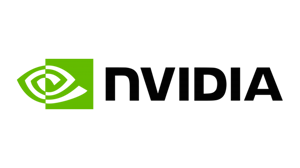
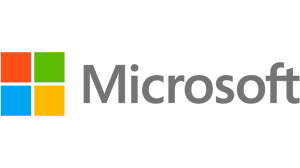
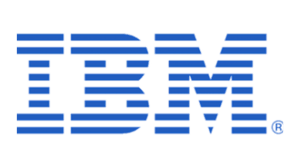
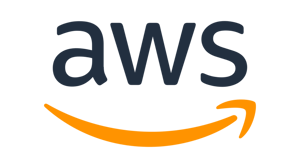
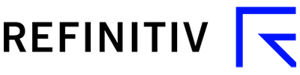
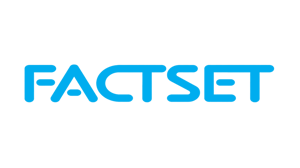
.png?width=300&name=UNIMORE%20(1).png)
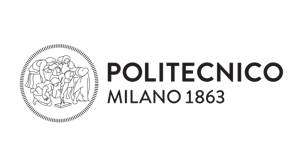
.png?width=300&name=UNIBO%20(1).png)
Explore the methodologies driving our AI model development and AI platform and learn more about our commitment to the construction of our innovative and robust processes.
TABLE OF CONTENTS
OUR METHODOLOGY
What is Axyon IRIS®?
Axyon AI Platform: Proprietary Auto-ML platform for financial time series. A highly automated process that explores and optimizes (i) a wide array of ML algorithms, including deep neural network models
(ii) their free parameters, (iii) hyperparameters, and (iv) the selection of input variables, and produces an optimized ensemble of models Tailored for financial time series data of any kind and frequency.
Axyon IRIS®: Data processing, inference and distribution engine of AI-powered investment strategies to institutional investors such as asset managers, hedge funds and corporate trading desks.
What kind of AI models are used in Axyon IRIS®?
Our focus is not on individual models, just like for car manufacturers the focus is not on fixing individual cars but rather on designing and implementing a high-performing, efficient and automated assembly line for producing cars. And this process evolves over time through incremental improvements. At the moment, Axyon IRIS® strategies are powered by ensembles of supervised learning models including neural network-based (both tabular and sequential) and tree-based models. First, a large number of candidate models is explored with a hyperparameter search algorithm. Secondly, such models are optimally combined to constitute an AI model ensemble that is used for historical (out-of-sample) prediction generation and is ultimately deployed to production.
What kind of data do we use?
We ingest data of different kinds and from multiple sources. The main data types used to create Axyon IRIS® datasets are EOD and intraday market data, fundamental indicators, macroeconomic indicators, related indexes or securities (e.g. commodities, VIX, FX, etc.), sentiment indicators extracted from news and social media, options data, and analyst forecasts. In very specific cases and with selected partners, we support the possibility of including proprietary indicators that our client might have developed - we currently do this with our two largest clients.
How is feature engineering performed?
Over time, we have created a feature store for financial time series problems that we maintain and continuously improve. Most of the data modelling work is carried out by our Quant Analysis team, whose work is integrated with our ML process, ensuring that every single feature can be computed with point-in-time data, is stationary, is expressed in a format that can be fed into supervised AI models, and it can be computed in live settings under time constraints.
How are feature selection & hyperparameter search performed in Axyon IRIS®?
The feature selection and hyperparameter optimization process employed to develop Axyon IRIS® models is part of our proprietary expertise and cannot be fully disclosed. The process involves an extensive exploration of the search space, whose dimension mainly depends on the dataset complexity (number of input variables) and modelling assumptions, which determine the set of AI models available to the search algorithm. In most real-world cases, a thorough exploration of this space is computationally infeasible (there may be more hyperparameter configurations than atoms in the universe), even with thousands of parallel compute hours on a cluster of GPU-powered computational nodes. Thus, we resort to a proprietary stochastic optimization method for optimizing hyperparameters and feature (sub)sets.
Are predictions in Axyon IRIS® explainable? How?
We believe that the explainability of a Machine Learning model can be as crucial as its performance in many business applications for many reasons (trust, regulatory, technical, ethical, etc.). Our AI platform natively supports a state-of-the-art technique called SHAP (short for Shapley Additive Explanations) to decompose each prediction into the contributions of each individual input variable. In other words, for each input variable we can compute a (positive or negative) contribution, and if we sum all these contribution we obtain the model’s prediction. As the typical number of input variables per model is in the range of 200~500 features, to simplify the interpretation of these contributions we can group together all features belonging to the same semantic “category” (e.g. all features related to the fundamentals of a certain stock) and compute their aggregated contribution. By doing this, we can explain a prediction in terms of a small number (5-6) of “feature categories”, e.g. corresponding to classical financial factors, thanks to the fact that SHAP values are additive.
HIGH-PERFORMING COMPUTING (HPC) INFRASTRUCTURE
Engineered with precision, our scalable HPC infrastructure supports our complex AI modelling development, ensuring our solutions are not only innovative but are also rendered with exceptional speed, robustness and accuracy.
Scalable and flexible DB-centric master-slave system architecture
A paragon of scalability and flexibility. Adapting to multifaceted AI modelling tasks, this architecture navigates through diverse computational demands, guaranteeing that your AI models are developed, tested, and deployed with superior efficiency, even as your data ecosystems expand and evolve.
Versatile Computational Resources
Our infrastructure leverages a spectrum of computational resources, both internal (on-premise) and external (cloud), including robust HPC clusters. This blend of diverse resources ensures that your AI modelling capabilities are not bound by computational constraints, offering an unrivalled platform where your data can be processed, analysed, and modelled with unparalleled depth and breadth.
Reliability and Data Security
Our models are entrusted to a system where reliability and data security are critical. Our focus on these elements ensures that the models and data are shielded with fortified security protocols and supported by a stable, dependable computational backbone, providing us with an unswervingly secure and reliable environment throughout our AI development journey.
Scalable HPC infrastructure
Our scalable HPC infrastructure is a commitment to our relentless innovation and improvement pursuit. Designed, developed, and scrupulously maintained, its capabilities have been further enhanced through intensive research projects undertaken in alliance with HPC specialists from CINECA. Explore our detailed whitepaper to learn more about our technological advancements and methodologies.
.png?width=300&name=header%20(1).png)